Aims and Scope
Real-world problems in the financial domain often involve complexity, noise, uncertainty and vagueness. It is difficult to handle such problems by the conventional analytical and numerical paradigms in addition to the need to handle large volumes of data at a proper speed.
Over the last decades, Computational Intelligence (CI) has been gaining traction in the domain as both a problem-solving strategy and a new analysis tool. Conventional methodologies in financial engineering, predictive analytics and business analytics have various limitations and usually need significant user/expert engagement in modelling and application. CI has established a new research track as well as a new stream of methodologies to be used in financial and economic analysis. Capacity of CI is much higher than traditional approaches such as econometrics and time series analysis. CI can handle not only expert knowledge but also knowledge extracted automatically from data with by utilizing sophisticated algorithms and computational instruments (e.g. neural networks, fuzzy logic, control systems).
One of the great challenges in conventional models and instruments in finance and economics is the volume of assumptions and corresponding requirements such as (but not limited to) normal distribution and convexity in problems like portfolio optimization, option pricing, algorithmic trading and risk management. After the 2008 financial crisis, it has been once again realized that traditional methods are not only incapable of recognizing irrationally elevated numbers, but these models are also very abstract considering the complexity of systems led by human judgment. There is an emerging need and a growing interest in CI solutions for financial engineering and economic analysis considering the gap arisen from simplifications and abstractions in traditional instruments such econometric analysis or financial methodologies with various assumptions (e.g. normality).
CI opens the way for completely new approaches like agent-based computational economics, in which market dynamics are modeled by evolving a large population of interacting heterogeneous agents. Thanks to the advances in areas like evolutionary computation or fuzzy systems we can now model autonomous agents who have the capabilities to adapt, to learn, to have chances and to strategically interact with others and the surroundings. Genetic programming or grammatical evolution open the possibilities for automatic trading rule identification, and artificial neural networks have major roles in domains like early bankruptcy prediction.
From a theoretical perspective, financial management and economics has established a powerful basis for understanding the monetary and economic phenomenon. By utilizing the capabilities of CI with proper integration of fundamental theories, a vast majority of common problems may be managed much efficiently and accurately. This special issue is dedicated to high-quality scholarly works and industrial solutions proposing original CI applications in finance and economics and/or addressing theoretical and practical challenges through solid empirical evidences.
Topics of Interest include
The aim of the special issue is to bring together the latest advances from both the theoretical and the application side at the intersection of computational intelligence in finance and economics. Authors are encouraged to submit high-quality original manuscripts in domains including (but not limited to):
Financial Engineering & Economics Applications
• Agent-Based Computational Economics
• Asset Pricing
• Business Analytics
• Algorithmic Trading
• Electricity/Energy Markets
• Big Data Finance & Economics
• Machine Learning for Financial Analysis and Forecasting
• Financial Data Mining
• Financial Engineering
• Financial Time Series Forecasting and Analysis
• Economic and Financial Decision Making under Uncertainty
• Artificial Immune Systems
• Portfolio Management and Optimization
• Market Simulation
• Risk Management
• Credit Risk Modelling
• Commodity Markets
• Pricing and Valuation
• Term Structure Models
• Trading Strategies
• Pricing of Structured Securities
• Asset Allocation
• Trading Systems
• Hedging Strategies
• Risk Arbitrage
• Sentiment Analysis and Behavioral Finance
• Low Frequency / High Severity Event Modelling
• Plasticity of Artificial Systems in Economics and Finance
• Exotic Options
Computational intelligence techniques considered include (but not limited to):
• Deep Learning and Artificial Neural Networks
• Evolutionary Computation
• Fuzzy Sets, Rough Sets, & Granular Computing
• Financial Data Mining
• Hybrid Systems
• Metaheuristics
• Support Vector Machines
• Swarm Intelligence
• Probabilistic Modeling/Inference
• Intelligent Trading Agents
• Trading Room Simulation
• Time Series Analysis
• Non-linear Dynamics
• Rules and XBRL for Financial Engineering Applications
• Semantic Web and Linked Data for Computer & Engineering Applications & Models
Submission Process
The IEEE CIM requires all prospective authors to submit their manuscripts in electronic format, as a PDF file. The maximum length for Papers is typically 20 double-spaced typed pages with 12-point font, including figures and references. Submitted manuscript must be typewritten in English in single column format. Authors of Papers should specify on the first page of their submitted manuscript up to 5 keywords. Additional information about submission guidelines and information for authors is provided at the IEEE CIM website.
Important Dates
Submission Deadline
December 31st, 2017
Notification of Review Results
March 15th, 2018
Submission of Revised Manuscripts
April 15th, 2018
Submission of Final Manuscript
June 15th, 2018
Special Issue Publication
Mid-October 2018
(November 2018 Issue)
Okan Duru
Nanyang Technological University, Singapore
Okan Duru is the Assistant Professor of Maritime Logistics and Finance at Nanyang Technological University, Singapore with a research focus on computational intelligence for predictive analytics in financial and economic phenomenon. He received his PhD degree from Kobe University, Japan on the long-term modelling of shipping freight rates. His research interests include fuzzy time series, dynamic model mining, learning algorithms for economic analysis with a special focus on transport economics and finance. He is also a member of Society for Computational Economics which is officially recognized by the American Economic Association (AEA). Okan Duru is particularly interested in intersecting topics between pure economics and computer science including economic forecasting, business analytics for economic analysis, predictive analytics and utilizing instrumental capacity of computer intelligence in common economic and financial problems. Okan Duru is the current vice-chair for the IEEE Technical Committee on Computational Finance and Economics.
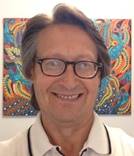
DBmind Technologies Inc., USA
Robert Golan is an Information/Rules Architect who is “hands on” with Service/Data Modeling while applying the Industry Standards. Robert is a specialist in the architecture, design, & development of Cloud based SOA Web/Restful Services for MDM/Data Warehouses with Business Intelligence and Business Rules/BPM integrations. Unstructured, Semi-structured, & Structured Data integrations with taxonomies, ontologies, vocabularies and Canonical/ Logical/Physical models are his specialty. The Data Sciences have been his focus since graduate studies where Robert has applied techniques from Machine Learning, Artificial/Computation Intelligence, Big Data, and Data Mining. Robert is a pioneer in the application of Computational Intelligence for Financial Engineering with emphasis on Advanced Algorithmic Trading strategies and Risk Management. Robert's research abilities coupled with his work experience, give him an outstanding ability to evaluate and apply new technologies and products. Robert has over thirty years of experience in designing, developing, and maintaining information technology systems while applying the needed information governance mechanisms. Project management, team leadership, and mentoring have been an integral part of Robert's project work. He has an extensive background with operating systems, communications, databases, and the internet. Robert’s domain knowledge cuts across the Financial, Pharmaceutical, HealthCare, Insurance, Energy, High Tech, and Agriculture industries. Robert Golan is the current chair for the IEEE Technical Committee on Computational Finance and Economics.

Carlos III de Madrid University, Spain
David Quintana holds Bachelor degrees in Business Administration and Computer Science. He has an M.S. in Intelligent Systems from Universidad Carlos III de Madrid and a Ph.D. in Finance from Universidad Pontificia Comillas (ICADE). He is currently and Interim Associate Professor with the Department of Computer Science at Universidad Carlos III de Madrid. There, he is part the bio-inspired algorithms group EVANNAI. His current research interests are mainly focused on applications of computational intelligence in finance and economics.
Coventry University, U.K.
Vasile Palade is a Reader in Pervasive Computing in the Faculty of Engineering and Computing and a member of the Cogent Computing Applied Research Centre at Coventry University. He previously held academic and research positions at the University of Oxford, UK (Departmental Lecturer in the Department of Computer Science), University of Hull, UK (Research Fellow in the Department of Engineering) and the University of Galati, Romania (Associate Professor in the Department of Computer Science and Engineering). He is the author of more than 120 papers in journals and conference proceedings as well as books on machine learning/computational intelligence and applications. His research interests include machine learning with various applications.